Exploring Machine Learning-Based Trading Strategies for Better Market Decisions
Introduction to Machine Learning in Trading
Machine learning, a subset of artificial intelligence, has become a transformative element in the domain of trading. By utilizing algorithms that can learn from data, traders can develop more nuanced trading strategies that account for complex market dynamics. This innovative approach is reshaping trading methodologies, allowing for the analysis of vast amounts of data in real time. The integration of machine learning into trading practices offers not only efficiency but also the potential for enhanced decision-making based on data-driven insights.
The traditional trading models primarily relied on historical price movements and fundamental analyses, often resulting in inadequate predictions in rapidly changing market conditions. Machine learning, however, empowers traders to go beyond these conventional methods. It enables them to detect patterns and correlations within large datasets that might be imperceptible to human analysts. Through supervised learning, machine learning algorithms can be trained on historical data, allowing them to refine their predictions over time as they adapt to new information.
The growing importance of machine learning in trading can be attributed to its ability to process and analyze data at unparalleled speeds. In an era where markets can be influenced by a myriad of factors — from economic indicators to global events — having a technology that can swiftly adapt and respond is invaluable. Additionally, as the financial markets become increasingly data-centric, leveraging machine learning provides traders with a competitive advantage to identify and exploit profitable opportunities.
Furthermore, the technology continues to evolve, incorporating advanced methods such as deep learning and natural language processing, which further enhance its application in trading. By combining these sophisticated techniques with traditional financial theories, traders can aspire to achieve better market decisions that are informed by empirical data rather than reliance on intuition. Embracing machine learning in trading signifies not only a shift in strategy but also a commitment to innovation in a fast-paced financial landscape.
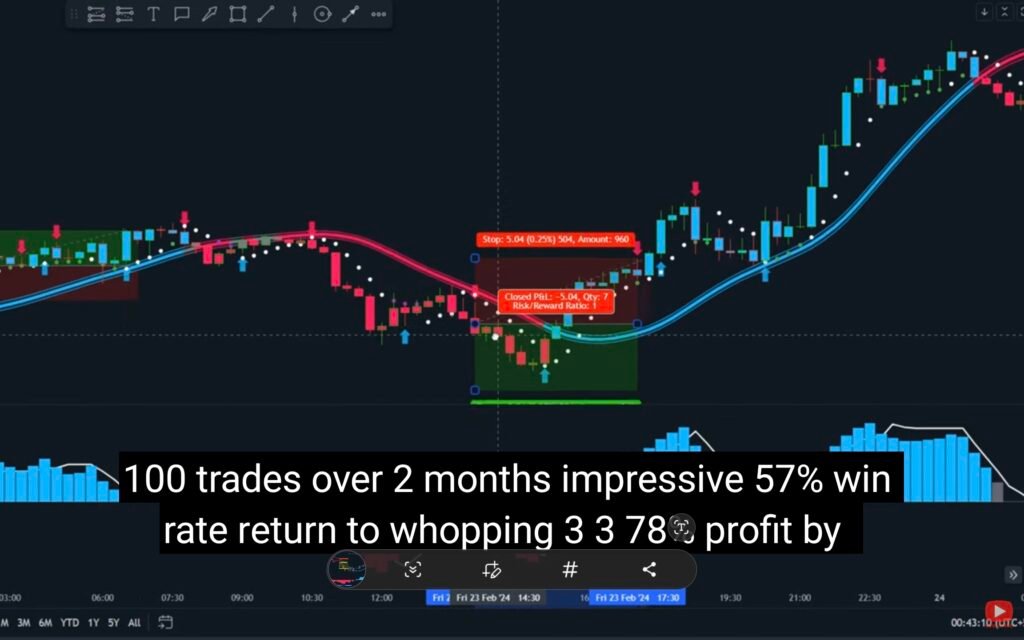
Overview of Trading Strategies
In the realm of finance, trading strategies are essential methodologies that guide traders in their pursuit of profitability within the markets. The essence of these strategies lies in their ability to inform traders about the best times to enter and exit positions. Three prominent trading strategies showcased in various resources include trend following, mean reversion, and arbitrage, each with distinct objectives and approaches.
Trend following is a strategy predicated on the observation that asset prices tend to move in persistent directions over time. Traders employing this method typically utilize technical indicators such as moving averages or momentum indicators to identify and confirm trends. The overarching goal is to capture gains by riding the momentum of established movements, allowing traders to optimize their entry and exit points based on the prevailing trend.
Contrastingly, mean reversion strategies operate on the premise that asset prices will revert to their historical averages over time. This method relies on statistical analysis to determine when an asset is overbought or oversold, leading to potential trading opportunities. Traders utilizing mean reversion seek to capitalize on price corrections by buying undervalued securities and selling overvalued ones, thereby enhancing their market decisions through a systematic approach to fluctuation analysis.
Lastly, the arbitrage strategy leverages price discrepancies between different markets or instruments. It involves simultaneously buying and selling an asset to exploit imbalances, ensuring almost risk-free profits. Traders engaged in arbitrage rely heavily on quick execution and sophisticated algorithms, often integrating machine learning techniques to identify and act upon fleeting opportunities rapidly.
These three strategies exemplify the diverse methodologies traders can employ to navigate intricate market dynamics. Understanding their unique goals and tactics lays the groundwork for a deeper exploration of machine learning’s role in optimizing these trading strategies.
Strategy 1: Supertrend, Parabolic SAR, and MACD
The integration of the Supertrend indicator, Parabolic Stop and Reverse (SAR), and the Moving Average Convergence Divergence (MACD) creates a robust trading strategy that aids traders in making informed market decisions. Each of these technical indicators plays a crucial role in identifying potential buy and sell signals, enhancing the overall trading experience.
The Supertrend indicator is famous for its ability to determine the overall trend direction in a market. By utilizing an average true range (ATR) as a measure of volatility, the Supertrend adjusts itself according to the price fluctuations. When the price fluctuates above the Supertrend line, it typically indicates a bullish trend, prompting traders to consider buying. Conversely, a price movement below the Supertrend suggests a bearish trend, resulting in potential sell signals.
On the other hand, the Parabolic SAR serves as a trailing stop indicator, providing dynamic price targets. It assists traders in evaluating entry and exit points by establishing a series of dots that indicate available support or resistance levels. A buy signal is generated when the dots are below the price, while a sell signal is indicated when they appear above the price. The combination of the Supertrend and Parabolic SAR indicators reinforces the decision-making process by offering multiple layers of confirmation, ensuring that traders are on the right side of the market trend.
Lastly, the MACD is useful for rendering momentum-based signals. Consisting of a MACD line and a signal line, it helps traders identify potential trend reversals. The crossing of these lines, particularly in conjunction with the signals from the Supertrend and Parabolic SAR, can yield powerful trading signals. When traders notice convergence, they gain valuable insight into potential market movements, allowing them to execute trades with increased confidence.
In conclusion, the strategic combination of Supertrend, Parabolic SAR, and MACD can substantially enhance trading efficiency. By understanding how these indicators operate individually and collectively, traders can forge a systematic approach for making better market decisions.
Strategy 2: Moving Averages and Swing Arm ATR
Moving averages and the Swing Arm Average True Range (ATR) are essential tools in technical analysis, often utilized to enhance the trading strategies and improve market decisions. By combining these two indicators, traders can define key support and resistance levels, which can be critical for identifying potential reversal points in the market.
Moving averages, specifically the simple moving average (SMA) and the exponential moving average (EMA), offer a smoothed view of price action over a defined period. The SMA is calculated by averaging the closing prices over a specified number of periods, while the EMA gives greater weight to recent prices, allowing it to respond more quickly to new information. These averages help traders identify the direction of the trend and potential price support or resistance levels. As traders observe interactions between the price and these moving averages, they can formulate insights into potential entry and exit points.
The Swing Arm ATR adds another layer of analysis by measuring market volatility. It is a modification of the traditional ATR indicator, enhancing its responsiveness to significant market movements. By calculating the range between the highest high and the lowest low, the Swing Arm ATR highlights periods of volatility as well as calm. When price approaches key moving averages while also displaying notable volatility, traders may interpret this convergence as a possible turning point. The combination of these indicators allows for more informed trading decisions, as it draws attention to places where price dynamics may shift.
Utilizing moving averages alongside the Swing Arm ATR can thus provide a strategic framework. This synergy helps traders recognize potential trends while understanding market volatility, fostering a more nuanced approach to navigating fluctuations in market conditions.
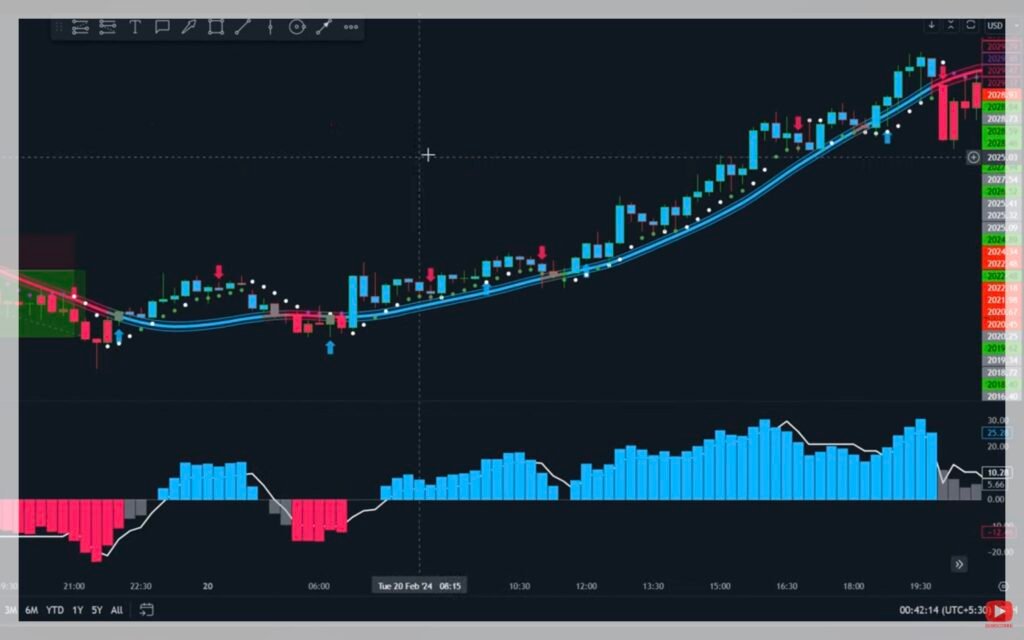
Strategy 3: Trader XO Macro Trend Scanner, MACD, and 200-Period Moving Average
The Trader XO Macro Trend Scanner is a robust tool designed to assist traders in identifying macro trends across various financial instruments. This strategy utilizes the scanner in conjunction with the Moving Average Convergence Divergence (MACD) indicator and a 200-period moving average, creating a comprehensive system for gauging market momentum and price movements. By combining these indicators, traders can identify potential buy and sell opportunities more effectively.
At its core, the Trader XO Macro Trend Scanner provides visual signals that help traders understand the overall market direction. It organizes data in a way that facilitates trend identification, ensuring that traders can spot both short-term and long-term trends accurately. When the scanner indicates an upward trend, it often signifies that bullish momentum is gaining strength, prompting traders to consider buying. Conversely, a downward signal may lead traders to consider selling or shorting the market.
The MACD is another critical component of this strategy that aids in confirming the trends identified by the Trader XO Macro Trend Scanner. This momentum oscillator calculates the difference between two moving averages, allowing traders to spot divergences between price action and momentum. When the MACD crosses above its signal line, it serves as a strong buy signal, while a cross below indicates a potential sell signal. When used alongside the 200-period moving average, which is a long-term trend indicator, the effectiveness of this strategy is significantly enhanced.
The 200-period moving average acts as a support or resistance level, providing context for price movements. When the price is above this average, the market is generally considered bullish, whereas prices below it indicate a bearish sentiment. Implementing these three elements—Trader XO Macro Trend Scanner, MACD, and the 200-period moving average—creates a structured approach that equips traders with valuable insights for improved market decision-making.
The Role of Market News in Trading Success
In the dynamic world of trading, the significance of market news cannot be overstated. Market news and relevant events play a vital role in influencing trader decisions and can dramatically affect market conditions, often creating fluctuations that technical analyses may not predict. As market participants make decisions based on a combination of data and intuition, staying updated with the latest news ensures a holistic approach to trading strategies.
Even the most sophisticated technical trading strategies can fall short if traders disregard the impact of current events. Economic indicators, corporate earnings reports, geopolitical developments, and central bank announcements can all lead to sudden shifts in market sentiment. For instance, a positive earnings report from a major corporation could drive stock prices upward, while political instability might trigger a sell-off across multiple sectors. A deep understanding of such market dynamics is vital for anyone looking to enhance their trading success.
Integrating real-time news insights into trading strategies is therefore essential. Traders need to leverage platforms that provide timely updates on market developments. Websites like Fast Bull offer a wealth of real-time information, enabling traders to remain informed about breaking news and significant market events. By being aware of the news cycle, traders can make more informed decisions, adjusting their strategies to mitigate risks and seize opportunities as they arise.
Moreover, understanding how different news types impact specific markets or assets aids in refining trading strategies. For example, traders specializing in forex might monitor economic reports closely, while those in commodities may pay more attention to weather forecasts and supply reports. Thus, recognizing the multifaceted relationship between news and market behavior is critical in executing successful trading strategies.
Importance of Backtesting Trading Strategies
Backtesting is an essential process in the development of trading strategies, particularly those that utilize machine learning algorithms. It involves simulating a trading strategy using historical market data to assess its potential effectiveness before it is deployed in live trading situations. This retrospective analysis allows traders and developers to evaluate how a strategy would have performed under past market conditions, which can be a fundamental step in refining their approach and boosting confidence in its future performance.
In the backtesting process, historical data is used to simulate trades based on specific entry and exit signals dictated by the trading strategy. This gives insights into various performance metrics, including profitability, risk-adjusted returns, and drawdowns. Commonly used metrics for evaluating backtested strategies include the Sharpe ratio, which measures risk-adjusted return; maximum drawdown, which assesses the largest peak-to-trough decline; and win/loss ratios, which offer insight into the percentage of profitable trades. Each of these metrics plays a pivotal role in understanding the potential viability of a trading approach.
Moreover, backtesting facilitates the identification of the strengths and weaknesses of a particular strategy. By analyzing the outcomes of simulated trades, traders can discover patterns that may not have been initially evident. For instance, they may find that certain conditions lead to better performance, while others result in significant losses. This leads to ongoing refinement and enhancement of the strategy. Adjustments based on backtesting results can help in mitigating risks, ensuring that the final strategy is robust, empirically validated, and better aligned with market realities.
In conclusion, thorough backtesting of machine learning-based trading strategies is critical for ensuring they are not only theoretically sound but also practical and effective when applied in real-world trading environments. By leveraging historical data to validate strategies, traders can make more informed decisions and enhance their overall market participation.
Risk Management: Protecting Your Investments
In the realm of trading, particularly when employing machine learning-based strategies, robust risk management is crucial for safeguarding investments and ensuring long-term profitability. Traders must recognize that while machine learning techniques can enhance decision-making, they do not eliminate risk; thus, implementing effective risk management practices is essential.
One of the primary methods of risk management is the use of stop-loss orders. This technique allows traders to set predefined limits on losses for each trade. By automatically closing a position once it reaches a particular loss threshold, stop-loss orders help traders avoid substantial financial setbacks. Additionally, setting take-profit levels can further bolster a trader’s strategy, effectively locking in profits when a desired price point is achieved.
Diversification is another fundamental risk management strategy. By distributing investments across various assets or sectors, traders can mitigate the impact of adverse movements in any single asset. Machine learning algorithms can well assist in identifying optimal diversification opportunities by analyzing historical correlations between different assets, ultimately leading to a more balanced and resilient portfolio.
Furthermore, position sizing is critical in managing risk effectively. Traders should determine the amount of capital to allocate to each trade based on their trading strategy, risk tolerance, and overall portfolio size. This disciplined approach ensures that no single trade can significantly impair the overall portfolio, reducing the potential for catastrophic losses.
A comprehensive understanding of market conditions is paramount for traders. Continuous education and analysis, supplemented by machine learning insights, can enable traders to better assess market dynamics and adapt their strategies accordingly. By adopting a disciplined approach to risk management, traders can enhance their resilience against market volatility, ultimately protecting their investments and improving decision-making outcomes.

Conclusion: Crafting Your Trading Plan
As we have explored throughout this discussion, machine learning-based trading strategies hold significant potential in enhancing market decisions. The application of these strategies allows traders to tap into sophisticated analytical techniques that can identify trends, predict price movements, and optimize trading outcomes. However, the effective utilization of these strategies requires a well-structured trading plan, tailored to the individual trader’s goals and risk tolerance.
When crafting your trading plan, it is essential to incorporate various machine learning methodologies suited to your investment style and objectives. Traders should consider the different models available, such as supervised and unsupervised learning, and assess which approaches align best with their market observations. Moreover, integrating decision trees or neural networks can facilitate more accurate predictions. Emphasizing thorough market analysis alongside machine learning will foster a comprehensive understanding of price dynamics.
In addition to employing machine learning techniques, a robust risk management framework is critical. This involves establishing clear parameters for loss limits, position sizing, and diversification to protect against market volatility. Continuous learning emerges as a vital component of any trading strategy, as markets are consistently evolving. Staying informed about the latest advancements in machine learning research and trading technologies can enhance your competencies as a trader. Engaging with educational resources, such as online courses and webinars, can provide valuable insights into refining your strategies.
Ultimately, taking a proactive and informed approach to trading not only enhances your capacity to make data-driven decisions but also equips you to navigate the complexities of financial markets. By synthesizing machine learning techniques with sound risk management and continuous education, you can develop a personalized trading plan that positions you for long-term success in the ever-evolving landscape of trading.